BCI2000
Type: Software,
Keywords: Adaptive neurotechnologies, Electrophysiology, System design & development, Neuromodulation, Brain-Computer Interfaces, Psychophysiology, EEG, ECoG, EMG
Resource ID: RRID:SCR_007346
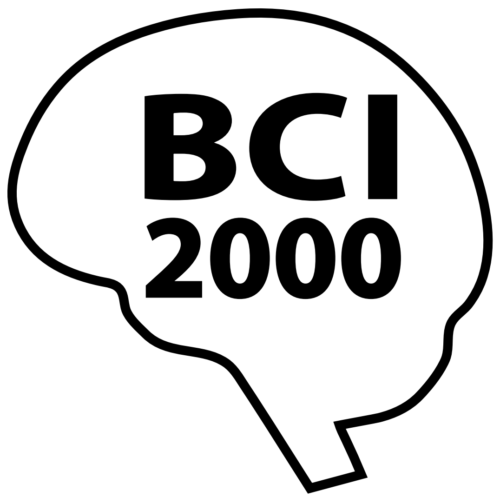
A general-purpose software platform for research and development of adaptive neurotechnologies
BCI2000 provides a portable open-source platform to implement the most common scenarios of adaptive neurotechnology research. BCI2000 acquires, synchronizes, and stores signals from a wide range of data acquisition systems, and translates these signals into useful outputs in real-time.
* Fully portable, configurable, and customizable ready-to-use platform for most common application scenarios in adaptive neurotechnology research.
* Acquires, synchronizes, and stores signals from a wide range of data acquisition systems.
* Translates signals into useful outputs in real-time.
* Synchronize data acquired from different sources (e.g., brain signals, other physiological signals such as electromyographic (EMG) activity, behavioral signals such as movement kinematics, audio or video) and at different sampling rates.
* Analyze multiple inputs rapidly to produce multiple outputs (e.g., visual feedback, electrical stimulation, prosthesis control); store all inputs and outputs for offline analyses.
* Facilitate the implementation and verification of all adaptive neurotechnology experiments planned in a particular laboratory.
* Facilitate the dissemination of scientific and clinical experiments across laboratories in different locations with varying hardware and personnel.
* Support an adaptive neurotechnology through its initial design and configuration, and the modeling and/or animal studies that define its characteristics and capabilities; support clinical studies to test hypotheses as to its ability to serve specific scientific, therapeutic, or diagnostic purposes.
* Produce data that includes all the information (e.g., recording and/or stimulation parameters) that is necessary for interpretation by personnel other than those individuals who collected the data.
* Acquire data from different inputs such as eye trackers or behavioral sensors (e.g., data gloves) from different manufacturers, and properly synchronize these data with physiological signals acquired at different sampling frequencies.
* Process different types of physiological signals (e.g., broadband activity, low-frequency oscillatory activity, evoked potentials, and single-unit action potentials).
* Visualize and store all its acquired data, together with a record of all events (timing and type of stimulation) that occur during operation.
* Realize fully automated neurotechnology systems that minimize the need for domain experts.
* Open-Source Code.
* Portable Experiments.
* General-Purpose Architecture.
* Highly Customizable.
* Intended for Research & Development.
* PC running Windows 10 or later.
* Supported data acquisition device.
* Belsten, A., Adamek, M., Brunner, P., Hardware Abstraction to Facilitate the Dissemination and Validation of Electrophysiological Experiments. EMBS 2020.
* Belsten, A., Adamek, M., Brunner, P., Overcoming Heterogeneous Hardware to Facilitate Dissemination and Validation of Electrophysiological Experiments. Society for Neuroscience Connectome, 2021.
* Adamek, M., Luczak, N., Mellinger, J., Refaey, K., Brunner, P., Evaluating the Closed-Loop Performance of Clinical Electrophysiology Recording Systems using BCI2000. Society for Neuroscience Connectome, 2021.
Peter Brunner, Associate Professor
Washington University School of Medicine in St. Louis
TEAM / COLLABORATOR(S)
Markus Adamek, M.Sc., Graduate Student, Washington University in St. Louis; Alexander F. Belsten, B.Sc., Research Assistant, Washington University in St. Louis; Hohyun Cho, Ph.D., Postdoctoral Associate, Washington University in St. Louis; William Engelhardt, Research Assistant, Washington University in St. Louis; Aysegul Gunduz, Ph.D., Scientific Steering Group Member, University of Florida; N. Jeremy Hill, Ph.D., Research Scientist, Albany Research Institute; Jane Huggins, Ph.D., Scientific Steering Group Member, University of Michigan; Dean Krusienski, Ph.D., Scientific Steering Group Member, Virginia Commonwealth University; Eric C. Leuthardt, M.D., Co-Investigator, Washington University in St. Louis; Nicholas Luczak, B.Sc., Research Assistant, Washington University in St. Louis; Kai J. Miller, M.D., Ph.D., Ph.D., Scientific Steering Group Member, Mayo Clinic Rochester; Daniel W. Moran, Ph.D., Co-Investigator, Washington University in St. Louis; Mohammad Amin Nourmohammadi, Ph.D., Postdoctoral Associate, Washington University in St. Louis; Nick Ramsey, Ph.D., Scientific Steering Group Member, University Medical Center Utrecht; James R. Swift, Ph.D., Postdoctoral Associate, Washington University in St. Louis; Jonathan R. Wolpaw, M.D., Scientific Advisor; Albany Research Institute; Tao Xie, Ph.D., Postdoctoral Associate, Washington University in St. Louis
FUNDING SOURCE(S)
NIH/NINDS U24-NS109103
NIH/NIBIB R01-EB026439